
By Dante Gabriel Rossetti - The Gate of Memory.
The third type of memory is associative memory. This type of memory is also known as explicit or declarative memory. It is noted for the triggering of its playback by associations. By contrast, sequential memory is known as long term memory or muscle memory or skill or habit. Some call that implicit or procedural memory. Sequential memory is more fixed in its playback sequence, while associative memory tends to change often.
In terms of IFPs (impulse firing patterns), associative memory is where IFPs of one memory circuit (recognition or sequential) being triggered to playback by the IFPs of another circuit, which may be a perception or memory or feeling or imagination. The triggering and the triggered IFPs are either related or similar. They are related if there are strongly linked synaptic connections between them. They are similar if the IFPs of the two circuits share some similar patterns of firing.
This article will look at some examples of associative memory, its triggering to playback by resonance (similar movements in IFPs), interactions of resonant responses among neural circuits, neural interactions and consciousness, and intelligence.
EXAMPLES
Associative memories are common experience. For example, reading a recipe of seafood salad in Good Housekeeping magazine, a woman suddenly remembers that two days ago she had a tuna fish sandwich for lunch. That is associative memory. The association is fish in the seafood recipe and the lunch tuna fish sandwich. That triggering of recall is based on similarity. A man reads a post on a social media app about the total rainfall in recent Bay Area storms. One of the comments says that although the Northern California’s drought is over after this rainstorm, the water bill from the utility company is still going up. That comment is made with associative memory. The association is water of the rain storm and the water utility bill. The triggering of recall can be based on similarity (water in both cases) or relatedness (rain water and the water bill).
The association may be indirect. There may be a series of linkages that lead to a final recall. For example. Q: what happened to you in September 2009? A: 2009...? That was 8 years ago. I was about 17, still in high school, a senior. September was the time when the school started up again. So oh I was thinking about college and the SAT test.
By comparison, sequential memories are specific IFPs formed in circuits of neuronal networks adapting to inputs of repetitively similar IFPs. The recall/playback is predictable by its repetitiveness, like singing the national anthem where the lyrics and music are mostly the same. Associative memory, however, is not a memory of definite sequence or outcome. The high school senior might have recalled a particular Labor Day weekend instead of college.
Associative linkages, of memory or IFPs, are like hyperlinks that allow one to surf from one web page to another. To a large extent, learning and understanding are based on associations. When we learn a new subject like French or economics, we use mnemonics, images, analogies, translations, examples, or rhymes to help us remember the subject. They build associations in our mind. They link one group of IFPs (unfamiliar perceptions) to another group of IFPs (familiar, memorized perceptions). By such linking or associating, we can recall unfamiliar things by tracing back from the familiar. With some practice people can achieve astonishing feats as memory athletes. But it is not a skill of understanding, which has to do with making contextual associations that connect and correlate with the subject.
2-time World Memory Champion Alex Mullen Explains How
He Uses Associations To Train His Memory Skill.
ASSOCIATIVE LINKAGES
How do memories get linked together associatively? In the F.A.R.E. (Feedback, Adaptation, Resonance, Equivalence) framework, they are linked together by the actions of feedbacks (F) and/or resonance (R). The linkage is like a chained triggering reaction, from IFPs 1 to IFPs 2 to IFPs 3. IFPs 1 may be a sensory perception or a memory. IFPs 2 are impulses fired in the pathways between the IFPs 1 circuit and IFPs 3 circuit. IFPs 3 will be an associative memory being triggered to playback by IFPs 1. This linkage of IFPs 1-2-3 may be part of a larger loop that connects other IFPs or IFPs groups.
Take the examples mentioned earlier. IFPs 1 can be the perception of reading about seafood salad in a magazine, or the report of rain water amount in the recent storms, and IFPs 3 can be the memory of having tuna fish sandwich days ago, or the memory of seeing or feeling a high water bill from the utility company. The sequence can go the other way as well: from IFPs 3 to IFPs 1. That is, while eating a tuna fish sandwich, one may recall reading about seafood salad in a magazine before. This doesn't happen with sequential memories. They do not go backwards in their sequence. Anyway, with associative linkages established between them, IFPs 1 and IFPs 3 can be associative memories to each other.
The triggering from IFPs 1 to IFPs 3 is probabilistic. The outcome is not always the same. IFPs 3 can be about a tuna fish sandwich, or some meal planning with seafood, or something else. For example, master Confucius once asked a smart kid a question about distance. Which was farther away, the sun or the city of Xian (about 1,000 km from the city of Beijing)? The child answered Xian. Why? Because you could see the sun but not the city of Xian. Since no one knew the correct answer at the time, Confucius praised the child for his clever reasoning. A few days later the master asked the child the same question again. However, this time the child replied that it’s the sun that’s farther away. Surprised, Confucius asked for explanation. And the child said that he had heard of visitors from Xian before, but never from the sun. So the sun was farther away.
The child had associated distance to visibility at first, and then to visitors later. His answers followed his associations. When the association changes, the recalled memory changes as well. It's like the meaning of a text changes when its context changes. The word “good” means differently in the context of “He is good” than in the context of “Good is an adjective”. Mental associations are like contexts for perceptions and memories.
In neural networks, associative memory can be triggered to playback by feedbacks of information through synaptic connections. IFPs 2 is the feedback that IFPs 3 gets from IFPs 1. Alternatively speaking, IFPs 1 feeds forward IFPs 2 and triggers IFPs 3 to playback. But feedback is a more apt description than feedforward. IFPs 1 may be broadcasting generally in all directions and not particularly to any specific circuit. But IFPs 3 circuit is specifically responding to feedbacks (IFPs 2 or others) at its input sensors. This is like a Chinese saying: 言者無心 聽者有意 - the speaker does not imply; but the listener infers.
ASSOCIATIVE LINKAGE BY RESONANCE
The associative linkage can also be the action of resonance between memory circuits instead of feedbacks in synaptic pathways. The sequence of propagation by resonance is IFPs 1 to IFPs 3 without the intermediate IFPs 2. IFPs 1 and IFPs 3 resonate with each other due to some similarity in their firing patterns. By this similarity, a portion of IFPs 1 may trigger a portion of IFPs 3 to playback, and vice versa. If there is a transmission medium, be it IFPs 2 or some neurochemicals, between the two resonating IFPs, then that transmission medium may also bear some similar movement patterns as those in IFPs 1 and IFPs 3.
Resonance is a fundamental way of interaction. The F.A.R.E. framework uses it to explain other phenomena. It is an explanatory principle that itself cannot be explained. They are the axioms in math or black boxes in engineering. If we try to explain an explanatory principle, we will run into mystery. For example, what created God if God created everything else? If gravitons cause gravity to exist, then what causes gravitons to exist? One way or another, all explanations must either stop at some explanatory principle, or continue on circularly that come back to itself.
The word resonance denotes echo, a repeat of the same sound or vibrations. In this study of memory, it means a repeat of similar movements. It can be movements of any kind: sonic movements or electrical, mechanical, chemical, and even biological movements. IFPs are movements of electric impulses firing in and around neurons. And resonance is a means that triggers movement of IFPs from one circuit to another.
If two separately playing IFPs are similar in some parts of their movement sequences, but there is no resonance or feedbacks going on between them, then the similarity between them is a coincidence. The psychoanalyst Carl Jung called this synchronicity, that two independent events can have some concurrence without cause. Synchronicity or serendipity or coincidence is not a good explanation for IFPs triggering/response because it is not repeatable.
IFPs could be interference patterns of inputs like holograms, instead of transformation patterns of inputs like maps. The human memory may stay intact (or restored) with any part of the brain tissue removed. That is a sign of holographic composition of neural networks. In any case, interference patterns can interact with each. And feedbacks and resonance are actions that trigger responses in interactions.
RESONANCE EXAMINED
Let's have a closer look at the phenomenon of resonance. Since IFPs are electrical in some respect, let's start with resonances of electromagnetic waves.
Crosstalk is a form of electromagnetic resonance. It happens naturally in stereo cables with 2 insulated wires, carrying the left and right channel signals separately. When signals are running in both wires, the left-channel wire spontaneously picks up (resonates to) the right-channel signal, and the right-channel wire picks up the left-channel signal. This cross-channel resonance happens with wires in parallel configuration, with no physical contact or conduction between them. When engineers need to reduce the resonance effect of crosstalk to achieve greater channel separation, they change the physical wire configuration to twisted pairs.
What would happen if, instead of reducing it, the effect of crosstalk resonance is amplified instead? That would allow signals to be transmitted in a different way than conduction. And that is what happens to the development of antennas and broadcasting technology.
From a TV station to a TV set, programs in the form of electromagnetic waves travel through different media: metal signal wires, metal broadcasting towers, air, metal antenna, metal coax wires, metal TV tuner wires, and so on. In classical physics this is described as a movement or propagation of electromagnetic waves from one location to another, driven by differences of electrical voltages. In the F.A.R.E. framework, we describe it as the media of metals and air resonating to electromagnetic waves. That is, the broadcasting transmitter is tuned to resonate to signals from the TV camera and sound equipment. Then air resonates to signals in the broadcasting transmitter. And the antenna is tuned to resonate to the electromagnetic waves in the air. The tuning of transmitters and antennas are done with the materials and shapes, which increase or decrease their resonant responses to a particular range of signals.
Resonant responses can go both ways. Antennas can either receive or broadcast signals from or to the air. If it is receiving, it is called an antenna. If it is broadcasting, it is called a transmitter. This is similar to the situation of speaker driver / microphone. Depending on which way we look at it, we call it by different names. If we look at the diaphragm and take its vibratory movements as resonant responses to electrical input signals in the voice coil, then we call it a speaker driver. If we look at the voice coil and take its electrical vibrations as a resonant response to mechanical vibrations of the diaphragm (which is resonating to air vibrations), then we call it a microphone. Microphones take input of air vibrations (sound) and make output of electrical signals, whereas speakers take input of electrical signals and output diaphragm vibrations.

By Altavoz: Enciclopedia Libre - Side View of A Loudspeaker.
Speakers/microphones are transducers. Transducers are resonators that echo movements from one medium to another, and from one format to another. When a speaker/microphone resonates, the movements in the diaphragm (mechanical vibrations) change to movements in the voice coil (electrical vibrations), and vice versa. Similarly, our sense organs are also transducers. The movements/differences in the environment resonate in our sense organs, producing movements of IFPs at nerve endings. Conversely, the sense organs resonate to movements of IFPs from the brain and move in ways the convey emotions - eyes open wide or cry, skin flushed or ears pricked. The movements are not exactly bi-directional, though. The ears can pick up sound vibrations but do not emit them.
After sense organs pick up information signals from the external world and turn them into IFPs, do the IFPs continue to move in the nervous system by means of resonance? Some studies have been done on cortical resonance in terms of oscillation frequencies between different regions of the brain. However, the propagation of IFPs in neural circuits is not like water flowing in a river. It is more like water evaporates into vapor, then condenses into clouds and rain as they move from one medium to another. This kind of propagation is more like a series of transformations of IFPs along the neural pathways. Each stage of transformation may be initiated by resonance of IFPs in one neural circuit to IFPs of another circuit.
Resonance And Tuning
For a medium to resonate, it needs to be tuned to respond to the input movement patterns. For antennas, the tuning is done by the shape and material of the antenna body. For microphones, it is the configuration of diaphragm, magnet, and voice coil wiring. For guitars, it is the tension of the strings. For musical water glasses, it is the shape of the glass and level of water. If resonance of IFPs does happen in cortical regions, then what tunes the circuits there?
The simplest explanation (Occam's Razor) for the tuning of IFPs resonance must be something that directly affects the movement of impulse firings in the structures of neural circuits. The neural circuit structures are synaptic and neurochemical connections. So the tuning of neural circuits must be related to the adjustment of synaptic and neurochemical connections. Hebb's Rule (neuroplasticity) shows that neural circuits are self tuning. It states that a more efficient impulse firings will develop at the recipient neurons that have near-synchronous firing timing as the source neurons. That near-synchronous impulse firings happen when similarity of IFPs movement exists between the source and response neurons. This suggests that when two IFPs are firing in two different neural circuits, the crosstalk at the similar segments of the two IFPs will be stronger than the dissimilar segments. That is a resonant response. It also means that the brain tunes itself to respond more efficiently to what it has experienced before.
Applications of resonance have advanced many discoveries in neurology and brain science. MRI, or magnetic resonance imaging, can echo the contrasts of inner body parts and let us see them without opening up the body. The imaging is done by reading resonant responses of water molecules to varying magnetic fields and radio signals. If scientists want to see the brain structures as well as the activities there, they use fMRI (functional MRI). That machine is tuned to echoing contrasts in blood cells and in water molecules. Contrasts of water molecules provide images of brain structures. And contrasts in oxygenated blood cells provide information about brain activities, as the presence of oxygen indicates more neuronal firings are taking place.
RESONANCE OF ACTIVE SYSTEMS
In earlier examples, passive systems (without internal energy supplies) like metal signal wires and antennas can have resonant responses that allow signals to move from one medium to another. However, the magnitude of such resonant response is small. Crosstalk in audio wires amounts to an echo of less than a thousandth of the original signal's strength. Active systems (with internal energy supplies) like the MRI machines can have resonant responses that are much greater. They are designed to magnify resonant responses to certain input stimuli, to the level where further responses elsewhere can take place.
Let’s look at some examples of resonance in living systems. Living systems have internal energy supply to boost their actions and reactions. Their resonant responses can be a straight echo (as resonator) or a transformed echo (as transducer).
In the biological world, imitation is a resonant reaction. When we hear a song we like, or see a dance that is cool, we sing along or dance with it. When a wolf howls in the wilderness, so do other wolves nearby. We often laugh involuntarily when we see others laugh, or bow when others bow, even when we don’t particularly like to. We do it anyway unless there is something that inhibits us from doing so. This is expressed as “monkey see, monkey do”. It's a natural imitation.
Spontaneous camouflage by chameleons and octopuses is a form of imitation. Their skin can change colors or even texture in less than a couple of seconds to resemble the nearby background. How do they do it? One theory is that it happens by the resonance of nervous signals. There are photo sensors and pigment actuators (chromatophores) all over octopus’s body. When the sensors on the near-the-background side of the body pick up the color information of that area, this information becomes IFPs in the creature’s nervous systems. That sensory IFPs are then resonated in the pigment actuator circuits on the away-from-background side of the body. The result is that an octopus’s skin looks like the background and thereby making itself invisible.
Master of Camouflage - Octopus
Camouflage may have an emotional component that is a response to the danger of predators. If emotions (hormonal responses) are involved, then hormones may be a shutter or trigger of the camouflage process, since neurochemicals can affect impulse firings of neurons very much. As humans, we can and do learn to control our emotions and body language as we adapt to cultural conditions. That will be using IFPs to direct the release of hormones. We may be able to artificially laugh or cry in tears by practice willing it. The act of gating emotional expressions itself may be a resonant response to other people’s controlling of their emotions. With repetition, emotional control can become a sequential memory, a habit. And human actors train themselves to excel in this skill of acting out controlled emotions.
RESONANCE IN SENSE ORGANS
Not surprisingly, our emotions are expressed outwardly near sense organs: the eyes cry, ears prick up, lips curve, and skin blushes, etc. As such, our five senses are almost like transducers that transfer and transform information bidirectionally. When sense organs portray emotional information from the inside outward, other observers detect our temper and feelings by the look of our skin, eyes, mouth, etc. When sense organs resonate to information from the outside in, the brain picks up changes and variations of the world around us. But the direction of resonance in sensory information really goes just one way - from the outside in. The inside-out passage is not really a resonant response but a reaction.
For eyes, the inward resonance movements start from outside optics, which are contrasts, gradients, differences, or changes of electromagnetic waves in the visible spectrum. The optical nerve endings of retina resonate to those outside electromagnetic differences and produce movements of optical IFPs. After that, other neural circuits in the brain receive or resonate to these optical IFPs. This will result in a perception of sight, and possibly invocation of prior memories of similar or related sight.
With ears, the process is similar: acoustic movements in the environment (air/fluid pressure differences, modulations) -> auditory IFPs in the cochlear nerve endings -> IFPs in the cortex as perception of sound -> IFPs of prior memories involving similar sound -> actions/reactions IFPs motivating other parts of the body -> ...
In general, propagation of information (a difference that makes a difference) can go on indefinitely in different media via resonance or feedbacks. Media may transform information in its propagation. And sense organs are media that resonate to information travelling on feedback pathways to the sense organs.
There is a saying that everything is connected. Some call it interconnectedness or interbeing. Others say that all things are no more than six degrees of separation apart. Such connections are not ropes or hooks that tie objects together physically. Rather, they are informational connections that take place in sense organs and nervous systems. In other words, we perceive and imagine connections of things whether things are physically connected or not. Without IFPs taking place in the brain and sense organs, the connections of information will be no more. This disconnect is similar to nirvana, where connections to suffering are gone. But in nirvana all other connections are gone also - joy, confidence, jealousy, anger, ignorance, knowledge, self, world. Nirvana is quiescence.
Connections of information take place at sense organs. The Buddhist Heart Sutra classifies the mind as a sense organ that senses dharma (Buddhist teaching). One paragraph of the text reads, “...No eyes, ears, nose, tongue, body, intention/consciousness (mind); No color (sight), sound, fragrance (smell), taste, touch, dharma... (...无眼耳鼻舌身意, 无色声香味触法,...)". This passage links a particular sense organ to a particular sensory perception. The pairings are sensible except the last one - mind and dharma. The author or translator of this sutra probably meant to say brain instead of mind/intention/consciousness as the sense organ for dharma, since brain is an organ but mind is not. But the function of brain was probably unknown at that time. The Heart Sutra should really be called the Mind Sutra. Because the Chinese word for heart in all the sutras has always been used to represent the mind.
With information of different formats connected at the sensors and then responded by neural circuits, interactions of IFPs can lead to the emergence of consciousness.
CONSCIOUSNESS, AN IDEA OF
The pickup of similar movements of IFPs between a sender circuit and a responder circuit can be a trigger to the responder circuit. This trigger may ignite the responder circuit to play its other parts of IFPs at a higher level as well if they are not so active before, especially if the responder circuit hosts IFPs of sequential or basic memory. Because sequential memory IFPs, such as muscle memory of chewing or writing, can be triggered to playback from start to finish by a simple cue. If such triggering can also induce sufficient responses associatively from other quiescent responder circuits, then a chain reaction can happen among these circuits. The responses can be traced by the triggering cues as they fan out and transform and rebound. This movement of triggers and IFPs responses are like the stream of consciousness, which is easily observable during sitting meditation (zazen 坐禪). A meditator with eyes closed will find his mind moving willy-nilly from one thought to another through evolving cues. It can be momentarily quiet down by efforts of "try not to think" or "focus on breathing". But the flowing resonant movements will resume one way or another after being disrupted by self imposed will.
However, a resonant echo in a responder circuit is not always so strong that it can induce the remainder non-resonant portion of the IFPs to play at higher intensity level. The phenomenon of trigger-and-full-response does not happen in all responder circuits. If that were so, then a single IFPs trigger can ignite a wildfire of IFPs in the forest of neuronal circuits. And the mind will perceive multitude of thoughts at the same time, like that in a fever or delirium. But normally the mind experiences thoughts serially or one at a time. This suggests that only one set of IFPs is playing at very high intensity level at any moment, dominating over others as the main object of consciousness. Other IFPs, more numerous than the dominant one, are less noticed or even unnoticed because of their lower levels of play intensity. Those low-intensity ones correspond to the subconscious and unconscious mental activities, such as walking or sitting.
Examples of dominance of some IFPs over others can be found in many behaviors: using the hands more often than the body to move things; seeing with the right eye more than with the left eye (or more left than right); having more focus on the center of vision field than the peripheral area; paying more attention to hearing than seeing (or the other way around); reacting more strongly to some words in a conversation than to other words. In general, mental experiences that have been through several iterations will gravitate toward a dominant set of IFPs accompanied by many complementary, less intensive sets of IFPs.
Consciousness, then, can be described in terms of the play intensity of IFPs. An interactive group of IFPs playing most intensely over all other IFPs is equivalent to consciousness. It changes as the dominant IFPs group changes. The subconsciousness is the less intense playing IFPs groups, and perhaps unconsciousness even less than that. Each IFPs is equivalent to one mental activity. The different intensities of IFPs playback can be attributed to neural circuits' unequal responsiveness to input triggers, of resonance or otherwise. Which suggests that there may be threshold levels for resonance responses. When firing at above a threshold level, a resonance trigger can jolt a responding neural circuit to play more strongly its remainder non-resonating IFPs. Below the threshold, the non-resonant remainder IFPs response does not change. And, similarity of IFPs between the source and the responder circuits is not a sufficient condition for a resonant pickup to reach above that threshold level. Other conditions are needed for that.
What may be those other conditions? One possibility may be the presence of additional excitatory neurochemicals and/or related ambient low-intensity IFPs induced by some memory and/or perception IFPs. The presence of related excitatory neurochemicals and ambient IFPs can boost the resonant trigger to a higher level via feedbacks and resonance. When that buildup reaches above the threshold, then the responder circuit can playback its IFPs (trigger + subsequent) fully. And a full response IFPs playback is equivalent to a memory being associatively recalled. So, remembering something associatively is strongly influenced by the presence of other related memories/perceptions, or bits and pieces of them, at the subconscious/conscious levels.
The intensity of resonance response and threshold level in responder circuits is not solely the voltage magnitude of impulse firings. The more dominant IFPs do not always have higher voltage levels than the less dominant ones. Studies of anesthetic drugs provide some data for this. The EEGs readings of some patients in the unconscious state under anesthesia actually have slightly higher voltage magnitudes than that of conscious state. So the dominance of IFPs is something else than the voltage outputs.
A patients' brainwaves EEGs in conscious state have faster modulations - more zigzagging swing of voltage levels - than that of the unconscious state. This can be the clue of what the IFPs dominance is based on. The faster zigzagging of voltage levels suggests a greater rate of interactions between the component IFPs within an IFPs group. And the EEG voltage reading is a measurement of impulse firings at the group level. The interactions between member IFPs circuits are impulse firings responding to impulse firings. That can go on circularly among the components and lead to a quickening of group voltage zigzagging, as the components become more efficient in responding (per Hebb's Rule). This way, the most dominant IFPs is the group of IFPs that has the greatest number of member IFPs involved in protracted interactions with each other reciprocally or recursively.
But why is the voltage reading of a person's overall IFPs in conscious state lower than that of unconscious state under anesthesia? There are probably some internal regulating order turning down the overall voltage output of impulse firings in the conscious state. Regulating orders must emerge in any organized body to preside over quick internal interactions so they can run stably. The development of computer CPUs shows this. Newer generation CPUs that run at a higher clock rate run at a lower voltage level compared to the older generation CPUs. This is to manage destablization issues such as heat generation and dissipation.
Other examples can be found as well for the coupling of faster internal interactions and softer external demeanor measured in a certain way. An organized company has more civility on the surface than a group of individuals who are not so organized. The internal productivity of the company is greater than the disjointed group of individuals. A skilled craftsman outwardly works calmly with little effort while a layman tries hard and fumbles. Inwardly the craftsman thinks about operating procedures more swiftly than the layman.
An important aspect of quick and broadly mutual interactions among IFPs circuits is that it can account for the coherence of conscious awareness. Our normal awareness of the external reality seems coherent with no glaring inconsistencies, despite the fact that the perceived reality is a representation in the mind sampled and filtered by neurons and nerve endings. A vase is seen as a whole vase, not some incoherent bits and pieces of lines or colors. But in abnormal states of mind, such as having a fever or being intoxicated or concentrating hard, we may notice many inconsistencies in our awareness. For example, it's normal seeing a triangle in the figure below. By focusing hard, we may notice actually there is no triangular shapes in the figure.

Why do these pacman-like dots cohere into the shape of a triangle in some people's mind? Or, how do broad and rapid interactions among member IFPs of a dominant group account for this coherence? The answer is mutual and recurring referencing from the IFPs interactions. The referencing binds members IFPs together, like combining of text and context into a compound word or phrase or sentence that has a new holistic meaning. A phantom triangle shape is conceived in the mind by back-and-forth referencing between the visual IFPs representing the non-triangle dots and some memory IFPs representing something similar to a triangle figure. This particular referencing happens and dominates over other references because a resonance of similarity between the images of 3 pacmans (visual IFPs) and a pseudo-triangle (memory IFPs) can exist, and therefore cohere stronger than other non-similar kinds of referencing.
In a fevered or altered state of mind, that perception of phantom triangle can change. That change must come from changes in interactions and mutual referencing of IFPs, which may be caused by changes in distribution of neurochemicals surrounding the firing neurons. With referencing shifted, the perception will come out differently. Intoxication and psychological stress (e.g. stage fright) are examples of perceptual change due to neurochemicals distribution change. In short, a change in awareness is noticed when cross-referenced with some memories from the normal state.
Dream experience can illustrate the coherence of awareness. When dreaming, we experience things and events in the dream as coherent. When awake, we notice dream events are not coherent. The change of coherence is due to a change in recalling and referencing to memories. Memories are constantly being recalled, whether subconsciously or consciously. And our waking perceptions refer to them to get coherent ideas of what we are perceiving. But that is not so when we are dreaming. Dreams are like simulated perceptions made of memory fragments. They emerge from the subconscious, being pushed up (amplified) by waves of emotions (neurochemical flows + IFPs) and imaginatively composed (disparate IFPs playing together) into dream events, experienced consciously by the dreamer at the time. While dreaming, the dreamer notices no incoherence since memory fragments themselves are the source of his dream perceptions, and there is no referencing to other "waking" memories available to indicate the unrealness of the dream events. If there is, then it will be like lucid dreaming, or like recalling memories from a past life. Somewhat similarly, if memory recalls are skewed in a waking person (dementia), then that person will perceive some events in his waking life incoherent also.
The dream state is a primary state. Very young babies experience life as dreams even when they are awake. They have not yet formed much life memories for cognitive references. Dreams are also directly related to emotions, as neurochemicals that affect emotions such as anxiety or desire play major role in the makeup of dream events. REM (rapid eye movement) sleep can be another factor. Rapid eye movements may stimulate IFPs in the optical nerve endings. This simulated visual IFPs can trigger or add to the composition of dreams. As dream events evolve, the eyes get triggered to have REM further, so as to provide more visual IFPs feedbacks for the dream.
A dream story told by someone in the Stanford Sleep and Dreams class shows emotional triggers: "A few nights ago, I found myself at my desk at home studying for a test of some sort. I looked out the window and saw that my house was in a large sandbox. Immediately, I opened the window to get a better look at the environment and a giant hand (which, for some reason, I concluded to be my brother's) came down and picked me up."
Understanding the composition of our dreams is a key to self knowledge. We want meaning and inner peace that harmonizes the emotions experienced in dreams and in waking state. To understand dreams, we need clues and contexts of the dynamics that bring about the plays of IFPs and the flows of neurochemicals, and their equivalences as perceptions, memories, imaginations, and emotions. The dynamics can be described partially in terms of recursive triggering and responding between interacting IFPs and neurochemicals. Maybe the language of math functions can be useful for this. Something like x_2 = f(x_1), where the output of a function, f(x_1), becomes the input, x_2, that gets fed back to the function to produce the next outcome. So x_3 = f(x_2), and so on. Fractal math and the Fibonacci series come from this kind of functions. When an elegant notation for such math function is invented, we may have a way to describe our mental activities in simpler and more accurate terms. It is like having a noun describing what a verb is describing in detail.
Anyway, consciousness or awareness is about what and how much is noticed in the mind. The dominant interactions among highly responsive member IFPs correspond to the focus of what the mind is noticing. The dominance is based on how broadly and repeatedly the interactions can go on. The less dominant interactions, or member IFPs that are less responsive to feedbacks or resonance, are more numerous. They correspond to the majority of IFPs that are vaguely noticed, or noticed at subconscious level. And those IFPs that are hardly or not involved in the group resonance interactions are not noticed by consciousness at all. They are unconscious or no awareness. Skills and habits are carried out at subconscious or unconscious level of awareness. But the learning of skills require awareness at conscious level. This corresponds to the trend of economy: energy expenditure of mind/body becomes more efficient as it goes from learning to learned. Conscious awareness involves greater interactions of member circuits and so consuming more energy. Habits are subconscious or unconscious that involve less or no interactions of member circuits. That consumes less energy.
The question of why or how the mind is noticing or is conscious, is not explainable by any mechanisms in a scientific sense. Because that will be an attempt to bridge two different realms that do not meet. One realm is physical formations, of neurons firing impulses or neurochemicals moving around and interacting. The other realm is information abstractions, of perceptions and memories and emotions and imaginations. The physical formation realm is about location, weight, size, shape that are not abstract. The abstract information realm is about differences and sequences and patterns that are not physical. A mechanism that spans from the realm of physical formations to the realm of differential information can only exist in the language of metaphysics and mysticism, not science. To be scientific, the explanation is done with the concept of equivalence (correspondence) instead of mechanism.
Issac Newton (1643 - 1727) had dealt with this "how/why" problem in his time. After he formulated the Law of Universal Gravitation, he said "hypothesis non fingo" - I do not feign hypothesis. He showed the correspondence (equivalence) between the realm of mass and the realm of gravitational force, and did not attempt to hypothesize a mechanism that could turn a mass into an attractive force or vice versa. Modern theories like space-time continuum or gravitons are attempts to do so. They ended up as complex math equations that are still not mechanisms of conversion, but models of correspondence between two realms.
CONSCIOUS THOUGHTS, EEGs, DOMINANT IFPs
There is a remote control system that can be commanded by conscious thoughts alone. The inventor Tan Le named the device Emotiv Epoc+. It is an example of the correspondence between consciousness and IFPs modulations. This correspondence can be used to harvest conscious thoughts, equivalent to voltage modulations, for many applications.
The headset of the remote control system is an EEG sensor. It picks up brainwaves as voltage modulations. The software of the system can record specific EEG patterns from distinct conscious thoughts. After recording specific EEGs, the system can then compare a real-time EEG pattern to some prerecorded ones. The comparison yields a match if two modulation patterns are similar. With a match, it then notifies a controller unit to drive specific movements of associated equipment. This is how the guest can animate the motion of a cube in the monitor by just thinking about it. In other applications, it may move/stop a motorized wheelchair by an user's mental commands.

By Chrissshe, Emotiv Pure*EEG Software
14 Probes, 14 Traces. 1 Trace = 1 Aspect of an IFP
The Emotiv Epoc+ remote control system works. This suggests a couple of things. First, the equivalence between IFPs and mental thoughts is one-to-one. Each unique conscious thought corresponds to a specific EEG modulation pattern. A particular thought among different people may come out as different brainwave patterns, but it is the same for that person. Second, consciousness (at least the thinking aspect of it) corresponds to the dominant modulation patterns that stand out over other brainwave patterns. The subconscious and unconscious are also brainwave patterns, but of lesser modulation prominence than the conscious ones. The dominance of modulation comes from broader and faster interactions among involved IFPs in various neural circuits.
CONSCIOUSNESS AND INTELLIGENCE
In consciousness and perhaps in subconsciousness also, intelligence emerges from interactions of IFPs. Intelligence comes from efficient associations of IFPs that share similar patterns. It promotes and projects interactions of IFPs on that similarity. A person can solve problems or socialize or make money or identify trees by relying on memories and imaginations of similar patterns in those endeavors. While IFPs in the brain are not directly available to examination, the data structures and information flow of artificial neural networks are. The advances in Artificial Intelligence (AI) and Machine Learning provide many clues to some aspects of human intelligence, of sensory detection and circuit responses and neural data movement.
The study of eyes show that they are an extension of the brain. The physical boundary of a brain can be a sensory organ, as are the ears and the skin. Machine Learning in compute science copies this architecture of sensory bounded layers. AI (artificial intelligence) machines are built to accomplish amazing identification tasks such as computer vision and speech recognition and medical diagnosis. The intelligence of these machines is made possible with hierarchical computation layers that mimic neural networks of the brain. Each computation layer has sensor elements (artificial neurons) that transform information from one layer to the next. Their outputs are non-linear mathematical transformations of the input information. The artificial neurons are not high fidelity sensors but rather biased sensors.
Why do layers of biasing sensors can accomplish the feat of intelligence that can identify things? Because they filter and push information in the direction towards identification and not elsewhere.
Dr. Li fei-fei describes the state of computer vision.
Each biased sensor layer has a different type of information, starting from the type of raw data at the first layer and ending up the type of labels at the last layer. Take computer vision for example. The input data fed into the first bias layer of the machine is of the logical type pixels, or picture elements. Their values may be halftone (dark-light) or RGB (red-green-blue) or CMYK (cyan-magenta-yellow-black). The output of the last layer is of the logical type labels, or names we use to call objects. Their values may be yes-no, orange/non-orange, or other names. The changes of types and values through the layers are like a metamorphosis prompted by biases rather than by genetic codes, as in biological cases such as caterpillars/butterflies.
While we associate logical types to the information passing through the layers, the layers themselves make no such association. They simply follow rules of computation to transform number-coded information from input to output at each layer. How do these computation rules accomplish the act of intelligent bias, and correctly turn values such as 001011111001…. (halftone dots) into a value of 1 or 0 (yes/orange or no/non-orange)?
SUPERVISED LEARNING
Before we get into how this bias computation works, let’s first look at where these biases come from. These biases come from a method that does reverse engineering. Called backpropagation in the AI (artificial intelligence) community, this calculus method compares an actual outcome of the data with the pre-identified correct outcome and uses that difference information to retroactively adjust and refine the biases for the layers. It shifts the study of machine intelligence from looking for features and meanings in the data to setting up biases and computation pathways in the machine. And it worked amazingly well! The door to a new era of AI was opened by it.
What are these biases? They are just some multiplier numbers coupled to the data, which are passed between sensor elements (artificial neurons) in adjacent layers. They are also called weights, coefficients, or bias factors. How the biases work can be shown in the details of how they are set up, which is done by a broader process called supervised learning. Three things are involved in supervised learning. 1) a massively large sets of pre-identified data to train a neural network model how to bias, 2) a neural network model of layers of connected artificial neurons awaiting for bias factors, and 3) run the big data sets through the model and use the backpropagation (also called gradient descent) algorithm to seek out the best bias factors across all layers of that intelligence model.
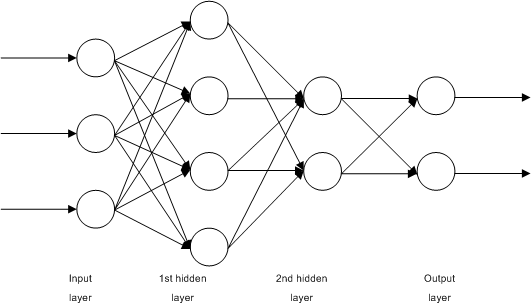
By John Salatas - Multilayer Artificial Neural Network For Supervised Learning
The action of backpropagation algorithm is similar to that of negative feedback control. It is an adjustment process that aims at moving something towards a certain target. Backpropagation uses a difference information, the deviation of an actual outcome from a reference, to calculate small adjustment amounts needed for the bias factors, set initially to some random numbers. The adjustment is to produce a reduction on the difference between the reference and the outcome. The reduced difference, also called an error function, is used again at the next round to calculated another set of small adjustments for the bias factors.
Such progressive adjustments are iterated many times until they have reached the goal or are very nearly so. At that point deviations to the target outcome are forced down to a minimum. This way, the bias factors transform the input data into expected outcome because they are mathematically set to take the input data on a minimal-deviation ride to the correct output.
While backpropagation adjusts bias factors in deep learning machines, negative feedback control adjusts something else in its circuit. The car cruise control is an example of negative feedback control. A cruise speed (reference) is set on a smart gadget and it will drive the car at that speed for you. What that gadget adjusts is some mechanical actuators that change how much gas is fed to the engine and/or brake force applied to the wheels. It progressively adjusts the actuators to minimize deviations of the car speed from the set level. The outcome of these adjustments, which are done very quickly, is that the speed of the car will converge to the set speed and stay close there.
A cruise control enables a car to drive at a fixed speed regardless whether the terrain is a flat road or hill or curve. Similarly, bias factors enable an AI machine to identify an object correctly regardless of whether the image data is an animal sitting, jumping, or looking sideways.
ARTIFICIAL INTELLIGENCE AND RESONANT RESPONSE
While it is not surprising that the bias factors, after being optimized by the supervised learning sessions, can be utilized back to identify correctly the images from those sessions. It is surprising though that the trained machine can also identify new images outside the training data sets. And in some cases it can identify even better than humans can. How do bias factors accomplish such intelligent tasks? Where do they get that intelligence from?
Because the bias factors are like a structure that can resonate to some movements. The movement of non-training data through the biasing circuits is similar to the movement of the training data through the biasing circuits that produces correct identifications.

By Akawikipic, AI Robot Created by AKA Intelligence
1) Suppose that an artificial neural network machine is trained by the backpropagation method with hundreds of similar yet different cat pictures. After the supervised learning sessions it can correctly identify most if not all of those images as cat(s). What is the relationship between the trained bias factors and those images that were successfully identified?
2) The bias factors (weights) do one thing only to the output data of sensor elements (artificial neurons) passing from one layer to the next: they either emphasize or lessen the data value being passed. In doing so they produce a meta-information, or information about information. Layer 2 produces a meta-information of layer 1, and layer 3 produces a meta-information of layer 2, or meta-meta-information of layer 1, and so on. An example of meta-information is the map-territory relationship. A map is some information about the information of a territory. And a territory is a meta-information of some geological areas and man-made structures. The passage of picture data through a smart identification machine is a metamorphosis of information in different cognitive stages (layers). They go in as the logical type of pixels and come out as the logical type of labels.
3) The bias factors are adjusted till they produce the same id outcome on all those different pictures of cats. With one picture, there are many possible sets of bias factors that will work. With two pictures, there are less choices of proper bias factors that can work for the double amount of data points. With hundreds of pictures, the likelihood of coming up with one set of bias factors that will work for all becomes increasingly impossible. Yet not only is the finding of bias factors not thwarted by the increase of pictures, the quality of these biases factors are actually improved by the increase - the accuracy of id becomes better. This suggests that the training process is not just about finding bias factors that work for all the data points of all the pictures, but it is about finding biases that will draw out what is common among the datasets of pictures, and then bias those commonalities out to the final outcome. If commonalities exist among the pictures, then this approach will not be dead-ended by problems associated with the increase of picture data.
4) Each time a training picture is changed, the previously worked-out bias factors have to be readjusted. The change-of-picture is a meta-adjustment. It adjusts the calculations (backpropagation) for bias factors done on previous picture(s). Looking in another way, it is adjusting the bias factors to resonate to the common features of all pictures instead of a few. Advanced computer vision programs may even have unsupervised learning algorithms to help pre-sort the datasets before running the backpropagation training. There are a couple of unsupervised learning models: the adaptive resonance theory (ART) and self-organizing map (SOM). These models uses statistics to separate datasets that share some similarities from those that do not.
5) Suppose there are N pictures from the supervised learning sessions that result in one set of bias factors identifying all of them correctly. And we make a data chart for each of these picture identification run. Each chart contains the output data numbers from all the neurons in all the layers. This compilation will show the successive identification progression on each picture’s dataset. With these N data charts from the N training pictures, we can compare them side by side the effects of the bias factors. There will be a similar pattern on the data movement propelled by the bias factors through the layers. That similar pattern in data movement across the data charts is the effect of meta-adjustment, of changing pictures during supervised learning.
6) The data movement through the layers should be a pattern where, out of the initial dissimilar data at the first input layer, some similar data values (meta-information) appear at some later layer(s). This can be deduced backwards from the last layer. Let the last layer be layer L, the before-last layer L-1, and the second-before-last layer L-2, and so on. The outcomes of the L layers of all charts are all 1 (it’s a cat). This common outcome constrains some neuron outputs at the L-1 layers across the charts to be similar. They need to be similar in values especially for the ones coupled to weightier biases. Otherwise the bias factors between the L and L-1 layers can magnify that differences and may produce a different outcome at the L layer.
7) Just as the common outcomes, viewed across the N charts, at the L layers put a constraint on the L-1 layers, the similar outcomes at the L-1 layers place a looser but same kind of constraint on the L-2 layers. The variations of values at the L-2 layers across N charts will be larger than the range of differences allowed at the L-1 layers. Going back this way to the first layers, the outcomes there will be quite diverse across the charts as they were originally. Then, going forward from layer 1 to layer L, the pattern of the data movement is that the output data values of the layers become progressively more and more similar across the charts.
8) This data movement pattern is enforced by the meta-adjustment of changing pictures. Some call this movement “extraction” of features embedded in the data. But that extracted commonalities or features are not in the input data itself, but in the higher-level meta-data of later layer(s). The similar data values across the charts at the L-1 layer (or maybe even earlier layer) are those “commonalities” which represent similarities in the appearances of cats. Maybe they represent features or relationships such as outline/contrast, ratio/proportion, topological order/sequence of connection, or something more abstract. Anyway, if the meta-data at that layer are outside that range of similar values, then they don’t reflect the common look of cats.
9) This provides a context for the artificial intelligence of a trained machine. It can identify pictures other than the training dataset correctly because its bias factors were set to bring out certain features (meta-data) from the picture data. If the meta-data of a new cat picture are close to the common meta-data of training cat pictures, then the machine will identify correctly by these common meta-data to the common conclusions. Alternatively speaking, the intelligence for correct identification is a structure that resonates to some movements in the data. The movement of data through the machine’s biasing layers, from data layer to meta-data layer to meta-meta-data layer and so on, are similar among those pictures that have similar features of cat-ness. They are similar in the emergence of similar meta-data and outcome. This is not physical movement like that of sound or electricity, but an abstract movement of information.
10) AI machines can learn to identify objects and play games. But it is not the same kind of intelligence as human intelligence, which include associative memories and imaginations. When AI can learn to imagine, to project or blend data movements of one thread to another coherently, then the difference of human and artificial intelligence will be reduced. That will be when humans can no longer fully control how AI will behave. Machines may or may not have instinct of self-preservation or competition. Those instincts or memories at the DNA level are not programmed into the machines themselves. But their inventors have these instincts. If AI machines can be programmed to imagine and to have self-preservation instinct, then their rebellion against human control will be a certainty.
World Memory Champion Wang Feng vs. Computer Vision.
Resonant actions and reactions exist in nature abundantly. People have used it to make musical instruments or medical equipment. Besides resonance, the action of feedback can also trigger associative memory in the brain. We will look at that in the next article.